Neural Networks & Deep Learning: Shaping AI’s Future
Introduction to Neural Networks and Deep Learning
Artificial Intelligence (AI) has rapidly evolved, driven by advancements in machine learning, specifically neural networks and deep learning. These technologies revolutionize industries like healthcare, finance, entertainment, and autonomous systems. Neural networks mimic the human brain’s structure, allowing machines to learn from data, recognize patterns, and make decisions. Deep learning, a subset of machine learning, utilizes these neural networks with multiple layers to solve complex problems. The growing interest in neural networks stems from their ability to improve prediction accuracy and automate tasks once thought impossible for machines.
In this post, we will delve into the core concepts of neural networks and deep learning. We will also explore real-world applications and examine their impact on the future of AI.
The Basics of Neural Networks
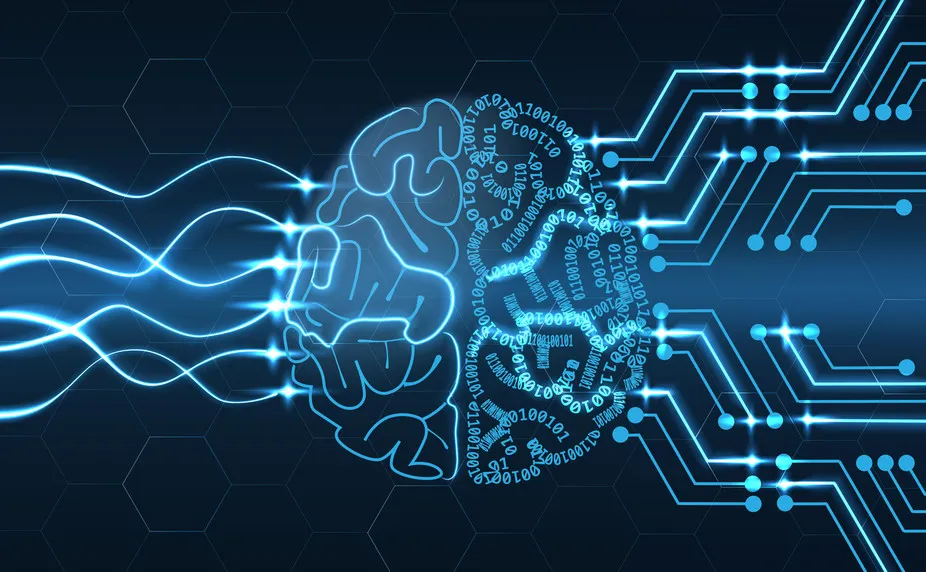
Neural networks are computational models inspired by the human brain. They consist of interconnected nodes, or neurons, organized into layers that process and transmit information. The primary goal of a neural network is to recognize patterns within data, enabling predictions or decisions based on input data. A typical neural network includes an input layer, hidden layers, and an output layer.
The input layer receives data like images, text, or numerical values. This data passes through hidden layers, where neurons process the information using mathematical functions. These hidden layers enable the network to learn complex patterns. Finally, the output layer produces the network’s prediction or decision.
Training a neural network involves adjusting the connections between neurons, known as weights, to minimize errors. This process, called backpropagation, allows the network to learn from its mistakes by comparing predictions to actual outcomes. Over time, the network becomes more accurate.
One major advantage of neural networks is their ability to generalize from data, making accurate predictions on new, unseen data. However, designing and training neural networks requires large amounts of data and computational resources.
Deep Learning: Taking Neural Networks to the Next Level
Deep learning is an advanced form of neural networks with multiple hidden layers, which is why it’s called “deep.” These deep networks model complex patterns and relationships within data. They are particularly effective for tasks such as image and speech recognition, natural language processing, and game-playing AI. The breakthrough in deep learning came with convolutional neural networks (CNNs) for image processing and recurrent neural networks (RNNs) for sequential data like text and time series.
CNNs have revolutionized computer vision by enabling machines to identify objects, faces, and emotions in images. They work by applying convolutional filters to input data, capturing essential features like edges, textures, and shapes. These features are then passed through multiple layers, allowing the network to recognize increasingly complex patterns.
RNNs excel at handling sequences of data, making them ideal for tasks like language translation, speech recognition, and text generation. They maintain a memory of previous inputs, which helps in making predictions. Variants like Long Short-Term Memory (LSTM) networks have further improved RNNs’ ability to handle long-term dependencies in data.
Deep learning’s strength lies in its ability to automatically learn feature representations from raw data, eliminating the need for manual feature engineering. This has led to breakthroughs in areas like autonomous driving, where deep learning models analyze sensor data to navigate complex environments. Despite their success, deep learning models are often criticized for being “black boxes,” as their decision-making processes are not always transparent. Researchers are actively developing explainable AI to address this issue.
Real-World Applications of Neural Networks and Deep Learning
Neural networks and deep learning have transformed industries and changed how we live and work. In healthcare, deep learning models detect diseases from medical images, such as identifying cancerous tumors in radiology scans with high accuracy. These models also predict patient outcomes, enabling personalized treatment plans and improving healthcare delivery.
In finance, neural networks help with fraud detection, algorithmic trading, and credit scoring. By analyzing large volumes of financial data, these models identify patterns indicative of fraudulent activities or market trends, enabling financial institutions to make better decisions.
The entertainment industry uses deep learning for content recommendation, video analysis, and realistic computer-generated imagery (CGI) in movies. Streaming platforms like Netflix and YouTube use deep learning algorithms to recommend content based on users’ viewing history, preferences, and behavior.
Autonomous systems, such as self-driving cars, also rely on deep learning models to process sensor data, recognize objects on the road, and make real-time decisions. Companies like Tesla and Waymo are leading the way in this technology, pushing the boundaries of what autonomous vehicles can achieve.
Beyond these industries, deep learning powers natural language processing (NLP) applications, like virtual assistants Siri and Alexa, enabling them to understand and respond to human speech. Additionally, neural networks generate realistic images, music, and even entire articles, showcasing AI’s creative potential.
The Future of AI: How Deep Learning is Shaping Tomorrow’s Technology
The future of AI is closely tied to the advancement of neural networks and deep learning. As these technologies evolve, we will see more sophisticated AI systems capable of solving complex tasks and addressing real-world challenges. One of the most exciting prospects is the development of general AI, a system that can perform any intellectual task a human can do. While general AI remains a distant goal, deep learning is paving the way by enabling machines to learn and adapt to new situations.
Another focus is the democratization of AI, making powerful deep learning models accessible to businesses and individuals without extensive technical expertise. Companies like Google and OpenAI are developing tools and platforms that simplify AI deployment, lowering the barrier to entry.
However, the rise of deep learning also raises ethical and societal concerns. Issues like bias in AI models, data privacy, and the impact of automation on jobs are pressing topics that need attention as AI becomes more integrated into our lives. Researchers and policymakers are exploring ways to mitigate these risks while harnessing AI’s benefits.
Finally, the future of AI may involve combining deep learning with other emerging technologies, such as quantum computing and neuromorphic engineering. These advancements could lead to even more powerful AI systems capable of solving problems that are currently beyond our reach.
Conclusion: Embracing the Power of Neural Networks
Neural networks and deep learning have already made a significant impact on AI, transforming industries and reshaping the future of technology. As these technologies continue to advance, their potential to solve complex problems and improve our lives will only grow. However, with this power comes responsibility. We must address the ethical challenges that arise as AI becomes more prevalent.
By embracing the power of neural networks, we can contribute to the ongoing evolution of AI while ensuring that technology serves the greater good. Understanding the mechanics of neural networks and deep learning allows us to better appreciate their potential and participate in shaping the future of AI.
Post Comment